Thomas Reutterer
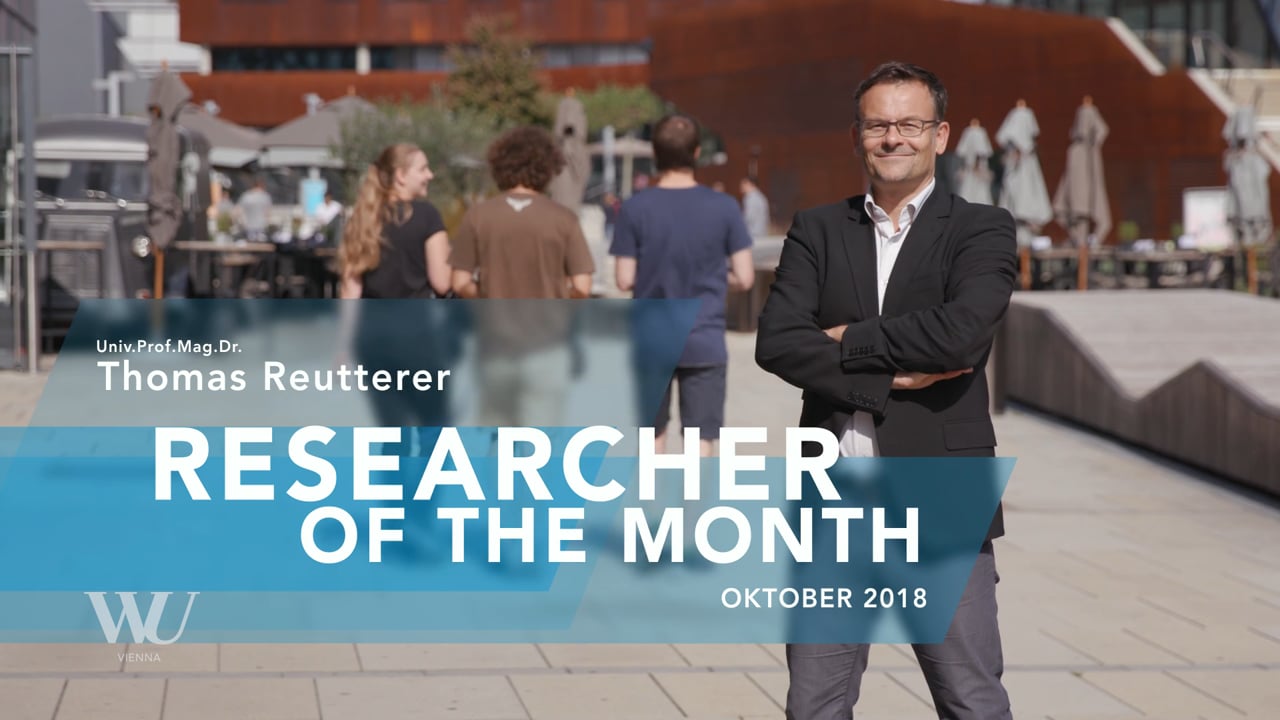
Thomas Reutterer
Researcher of the Month
What’s the value of my customers, and how long will they stay?
Not all customers are equally valuable to an organization. Building customer relationships with a high long-term value is extremely important. In his research, Thomas Reutterer from WU’s Institute for Service Marketing and Tourism is looking at how we can measure and predict the imaginary long-term value of customers, a concept called customer lifetime value. Professor Reutterer’s work shows that the regularity of customer interaction is a crucial factor. If regularity is taken into account, it is possible to make much more accurate predictions of how much value a customer will bring to an enterprise.
Customer lifetime value, i.e. the total value of a customer’s business with a company, does not only depend on how much time passes until a customer returns to buy another product or service. In more accurate terms, customer lifetime value refers to the amount of economically relevant interactions a given customer is expected to have with a company in the long term. How many purchases, service requests, and recommendations will the customer make? How long will the relationship with that customer last? When is the customer likely to move on to another company? WU Professor Thomas Reutterer, head of the Institute for Service Marketing and Tourism, is working to answer all of these questions, and to answer them as accurately as possible. Reutterer is working with Michael Platzer from Mostly AI and a team of data scientists to develop and test statistical models for predicting customer lifetime value. In a further step, these models can also be used to identify relevant factors that influence customer lifetime value. Professor Reutterer’s research focuses on non-contractual customer-firm relationships, where companies can never be quite sure if a person is still an active customer or has already moved away.
In customer interactions, regularity is key
Some crucial factors have already been established in marketing research: recency (time passed since the last interaction with the customer), frequency (the number of purchases a customer has made), and monetary value (the amount of money a customer has spent on the transactions). In addition to these known factors, Thomas Reutterer has identified another key dimension: regularity. “Our research clearly shows that if we account for this small piece of additional information – the regularity of interactions between the customer and the company – we are able to make much better predictions of churn, i.e. the moment when the company loses the customer. This allows us to draw much more reliable conclusions about the future value of a customer,” Professor Reutterer explains. His research results have been used to develop an open source software toolbox for predicting customer behavior. This software is designed to help companies make better predictions and improve their customer relationship management activities.
Stepping up the game with machine learning and deep learning
In addition to data on regularity, companies today also have access to many other types of information related to customer interaction patterns. Professor Reutterer and his team are currently working to integrate these types of information into their models. “We can assume, for instance, that customers’ activities on social networks or their preferences for certain products or services can tell us something about their customer lifetime value. And we can also gain valuable information by looking at how customers responded to previous marketing activities. We are currently working hard to integrate further types of data into our models for predicting customer lifetime value, using our experiences so far as a basis. In our current work, we’re also using machine learning and deep learning methods, which has proven to be a very promising line of research,” Thomas Reutterer explains.